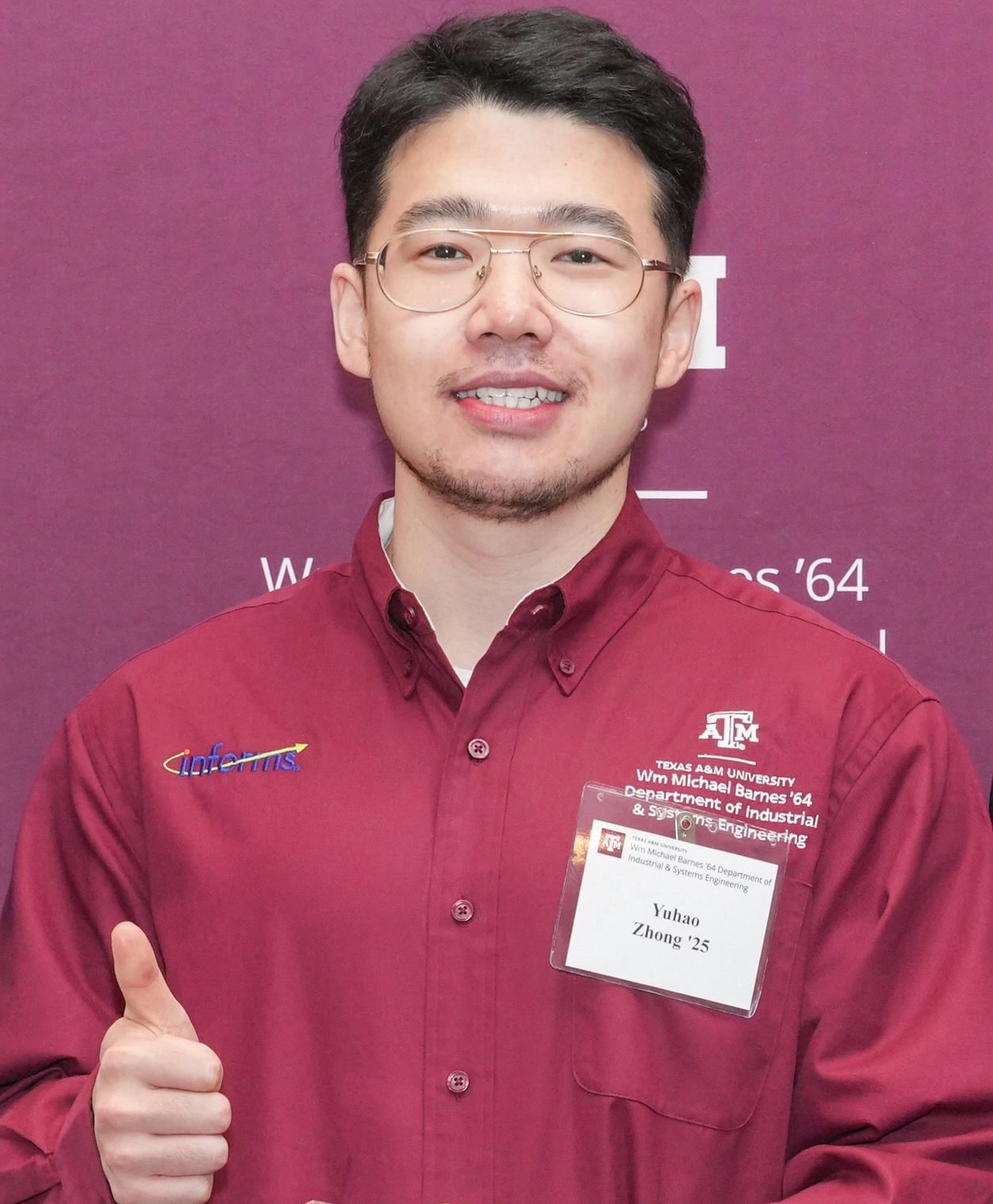
Yuhao Zhong (钟毓豪)
Ph.D. Candidate, advised by Prof. Satish Bukkapatnam
Wm Michael Barnes '64 Department of Industrial and Systems Engineering, Texas A&M University
Assistant Professor (Starting Fall 2025)
Grado Department of Industrial and Systems Engineering, Virginia Tech
[hirobin_zhong at tamu dot edu] [CV] [Google Scholar] [Linkedin]
My research focuses on advancing data science methods and harnessing sensors, machines, and human capabilities to address fundamental challenges in quality, safety, and performance assurance, and to facilitate knowledge discovery—primarily in manufacturing processes and systems, and more broadly in Industry 4.0 and 5.0 contexts.
I'm actively seeking self-motivated PhD students. If you're interested, please email your CV to [email protected].
If you're a current VT Master’s/Undergrad student interested in exploring research with us, also feel free to reach out.
Current Research Areas
- Explainable AI, generative AI, computer vision, and statistical methods
- Process-structure-property (PSP) knowledge discovery and uncertainty quantification
- Anomaly detection/localization and prognostics
- Human-robot collaboration
Latest News
-
05/2025 -
Cheers! Our team has been selected as a finalist in the 2025 IISE QCRE Data Challenge.
Kudos to the main contributors: Shashank Galla, Haopeng Tian, and Abhishek Hanchate! I will represent our team and present our results at the IISE Annual Conference. See you there!
-
04/2025 -
Cheers! Our paper "When textures deceive: Weakly supervised industrial anomaly detection with adapted-loss CycleGAN" has been accepted at the 2025 IEEE/CVF CVPR Workshop on Visual Anomaly and Novelty Detection (VAND 3.0).
Congratulations, Tapan Ganatma Nakkina!
-
03/2025 -
Cheers! Our paper "Efficient screening of rare large pit anomalies on polished surfaces using a minimalist sampling scheme" has been fast-tracked to the SME Journal of Manufacturing Processes and won the NAMRC Outstanding Paper Award in Manufacturing Processes!
I will be presenting this work at the Student Research Presentation Competition during SME NAMRC 53. See you there in June!
Selected Papers
-
EBLIME: Enhanced Bayesian Local Interpretable Model-agnostic Explanations
Authors: Yuhao Zhong, Anirban Bhattacharya, Satish Bukkapatnam
In: arxiv preprint, 2024
TLDR: A Bayesian regularized approach to locally explain black-box model and quantify the explanation uncertainty more accurately. It can also be applied to defect segmentation and knowledge discovery.
-
Detecting Anomalous Robot Motion in Collaborative Robotic Manufacturing Systems
Authors: Yuhao Zhong, Yalun Wen, Sarah Hopko, Adithyaa Karthikeyan, Prabhakar Pagilla, Ranjana K. Mehta, and Satish T.S. Bukkapatnam
In: IEEE Internet of Things Journal, 2024
TLDR: External IoT surveillance cameras combined with marker-based pose estimation and LSTM to track fast-moving robot and detect anomalous robot motion based on risks in human-robot collaborative industrial environment.
-
Identifying the Influence of Surface Texture Waveforms on Colors of Polished Surfaces using an Explainable AI Approach
Authors: Yuhao Zhong, Akash Tiwari, Hitomi Yamaguchi, Akhlesh Lakhtakia, Satish T.S. Bukkapatnam
In: IISE Transactions, 2023
TLDR: Consolidating LIME local explanations into consistent global knowledge using a query-by-expert algorithm. We identified the influence of surface textures on the colors of stainless steel 304 surfaces polished via Magnetic Abrasive Finishing. The discovered physical knowledge was validated through confirmatory experiments and blind tests.
Selected Awards
- Outstanding Paper Award in Manufacturing Processes, North American Manufacturing Research Conference (NAMRC), 2025
- U.S. Senator Phil Gramm Doctoral Fellowship, 2024
- Fox Graduate Fellowship, 2024
- Texas A&M Institute of Data Science (TAMIDS) Student Ambassador Scholarship, 2023 & 2024
- Winner, IISE Data Analytics & Information Systems (DAIS) Student Best Paper Contest, 2023